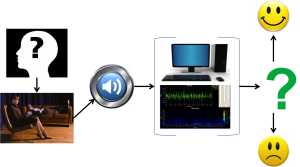
1. Can the analysis of prosodic cues be used as a reliable diagnostic tool?
2. Are these prosodic cues mimicked by the listener, i.e., can you detect a patient’s depression using prosodic cues from the clinician
Approximately 20 million people suffer from psychiatric depression. This disease accounts for over 40,000 suicides in the U.S. annually, but only half of MDD indications receive adequate treatment consistent with the American Psychiatric Association’s guidelines. A major contributing factor to this problem is the alarming rate of MDD misdiagnosis: 25%. The Hamilton Rating Scale for Depression (HRSD), the gold standard in depression assessment, is used as a benchmark for quantifying treatment efficacy. In it, the diagnosing psychiatrist makes a subjective judgment as to the severity of clinical symptoms based on information provided by the patient and his/her own assessment of the patient’s verbal cues and body language.
In an effort to improve diagnostic accuracy, a computerized algorithm relying on digital processing of patient vocal charactersits to assess depression state was created. The algorithm was designed using audio recordings of Hamilton Depression Interviews. Recordings were separated by speaker into tiers using Praat, which then extracts key acoustic metrics (fundamental frequency, pause duration, and intensity variation). An algorithm to analyze these cues was designed using Matlab, employing a K-nearest neighbor machine learning algorithm. This algorithm produces a predicted score within a confidence interval and was able to correctly predict the Hamilton Score assigned to the participant >80% of the time.
This algorithm was designed with former students Michael Borochin, Vikram Iyer, and Anand Vemuri of the University of Pennsylvania
Clinical Application
•This algorithm could greatly increase diagnostic accuracy of depression, thereby improving the chances of successful treatment. The algorithm in its current form is fairly user friendly, but can be time consuming.
•Future study could aim to create automatic software to analyze recordings with little user input that could be made widely available to practicing clinicians.
Comments are closed.